The implementation of machine learning into fraud prevention systems has increased merchants’ fraud fighting abilities to, quite literally, inhuman levels. And with global losses to payments fraud expected to hit $206 billion by 2025, the need for systems that can tackle the problem at scale is clear.
In this climate, what role do rules-based systems play in fraud prevention? And do they have utility above and beyond what a machine learning system provides?
In short, yes. The ever-evolving nature of the fraud threat in the online economy demands a multifaceted approach, and the combination of machine learning and fraud rules is the one-two punch that will give you the edge in this constant battle.
In this article, we make the case for fraud rules, explain how fraud rules engines work, and describe how powerful they can be when used in conjunction with machine learning.
What is a fraud rules engine?
A fraud rules engine uses a simple ‘if X occurs, it triggers Y’ system to detect and block potentially fraudulent transactions. At their most basic, merchants can use preset rules to, for example, route transactions with particular characteristics for more stringent security checks. At their most sophisticated, they can be customized to meet very specific risk appetites and business goals.
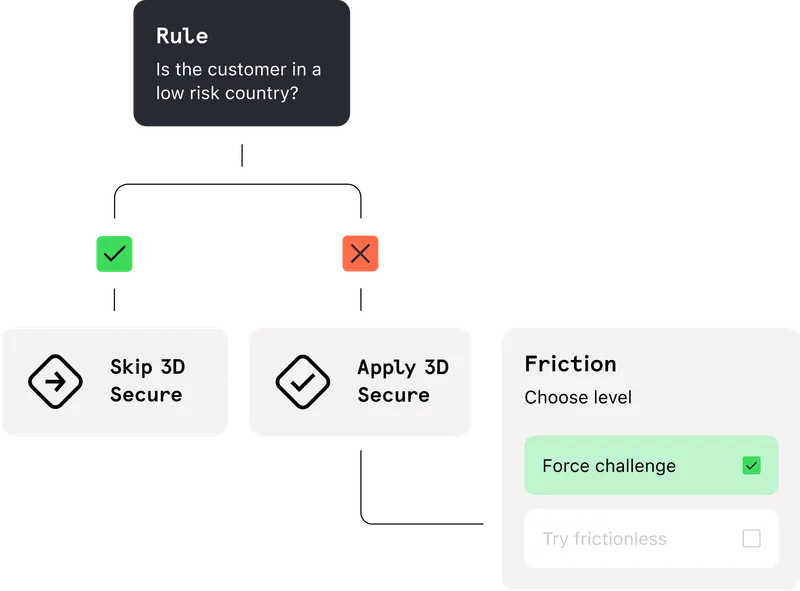
The main difference between a fraud rules engine and machine learning is that, with the former, you have full control and clarity over how data is being used to make decisions.
How does a fraud rules engine work?
The goal of a fraud rules engine isn’t just to detect and prevent fraud, it’s to do so while increasing acceptance of, and improving user experience for, legitimate customer transactions. Because, while it’s important to block criminal activity, an overzealous fraud engine risks damaging your revenue and your relationships with your customers.
That’s where the rules come in handy: allowing you to define exactly what a high-risk transaction looks like for your business in contrast to a genuine customer payment. For example, you could create a rule that sends any payment from a particular location or that surpasses a certain monetary value for further authorization.
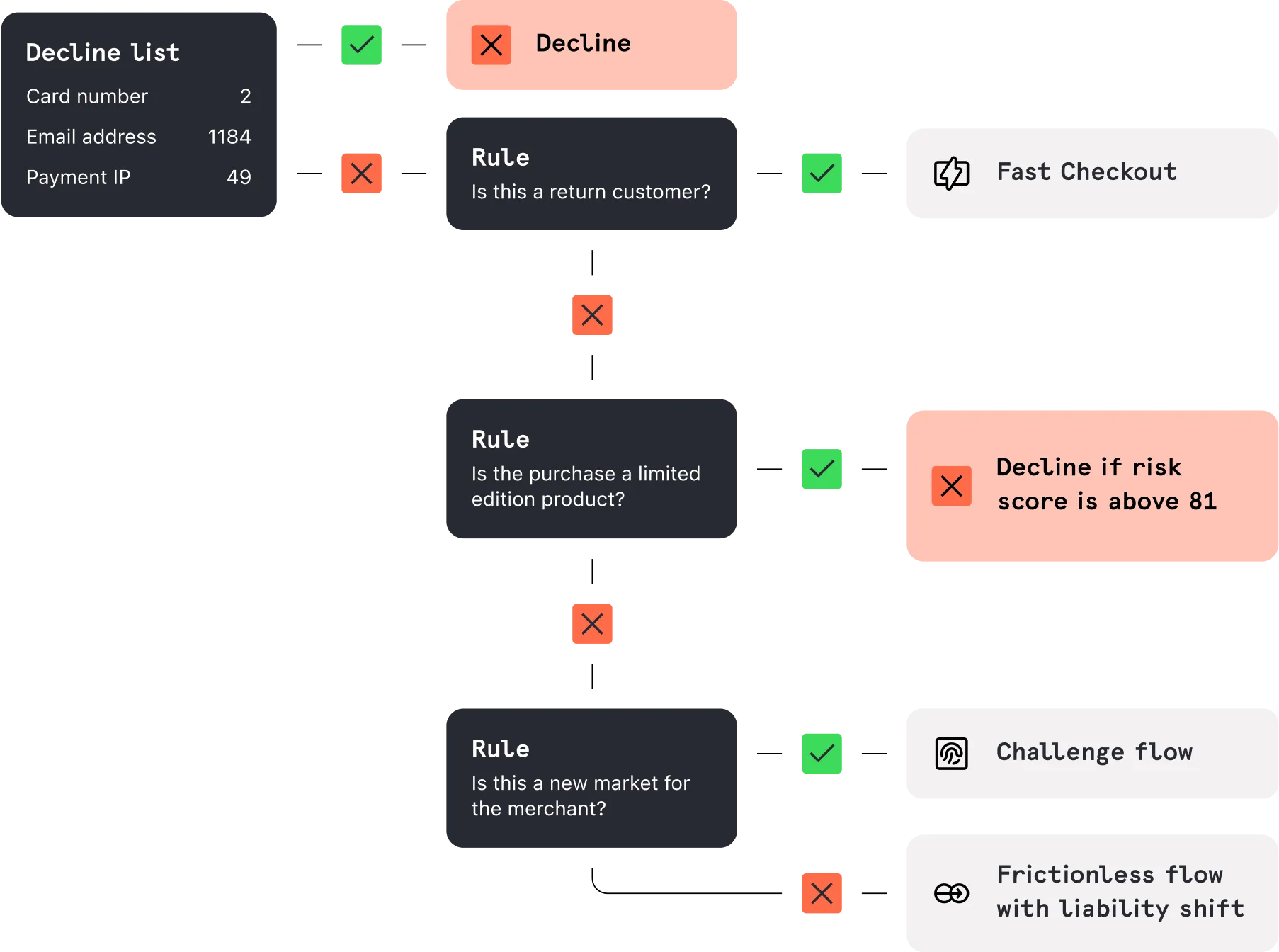
To be effective at this, fraud rules engines combine the following tools:
- Trust and deny lists - you can upload a list of key customer attributes (card numbers, BINs, email addresses, phone numbers, payment IP or email domains) to your rules engine and indicate whether they should be trusted or not. If trusted, the payment will be automatically approved; if not trusted, it will be automatically declined
- Rules engine - you have full flexibility to create rules that match the specific risks your business faces. These rules can be applied at either the pre-authorization stage (to automatically route a transaction for approval, denial or 3DS) or the post-authorization phase (to void, capture or flag a transaction for manual review that has already been through 3DS). You can also create complex rules with weighted scores, and multiple arguments and properties
- Machine learning - machine learning allows your rules to be used at scale. These models have been trained on billions of transactions across the payments network, each of which is given a risk score from 0 to 100 - an assessment of how likely it is to be fraudulent. These scores are used to define thresholds, which, if breached, result in the payment being automatically approved, denied or sent for 3DS
- Testing and analytics - your rules engine should also allow you to test and analyze the rules you create in order to, firstly, check the effect of new rules before implementing them, and secondly, assess the performance of your risk strategy after implementation. Testing can use either historical data or live traffic to simulate how a new rule will function without any negative impacts. Analytics can be used to identify fraud patterns, visualize the entire lifecycle of a transaction, understand performance over time and, ultimately, refine your strategy
Fraud rules vs. Machine learning: Which is more effective in fighting fraud?
As you can see, both fraud rules and machine learning rely on specific scores, thresholds and characteristics to decide how to route transactions - but which is better at actually preventing fraud?
Both play an important role. Machine learning acts as the first line of defense, capable of analyzing a vast quantity of data 24/7 and automatically triggering actions without the need for human intervention. ML models can start learning how to protect your business straight out of the box, but have little scope for customization.
In contrast, fraud rules do require you to dedicate time and resources (you may even need to hire a full time data scientist) to creating effective conditions and thresholds and measuring their performance, but they also give you much more control and oversight of your risk strategy.
Benefits of using a fraud rules engine alongside machine learning
In truth, fraud rules and machine learning are most effective when used in combination with each other.
Lets you see what is happening with each transaction
Machine learning is highly effective behind the scenes, but it is a black box. That means that, while you might know the inputs, you don’t get to see how the algorithm combines variables to make a decision. In contrast, with a rules-based system, you understand exactly which criteria were used to block or deny transactions, and you can review the journey of each transaction through your payment system. By using both, you benefit from the efficiency of machine learning as well as the transparency of a rules-based system.
Gives you more control
With a rules-based system, you define the rules and set the thresholds, which gives you full control over your risk strategy. This makes it easier to spot limitations, measure performance, and iterate and improve your rules over time. This can also lead to a higher degree of accuracy when routing transactions, whereas machine learning systems can sometimes misclassify data, which could lead to false declines and damaged customer trust.
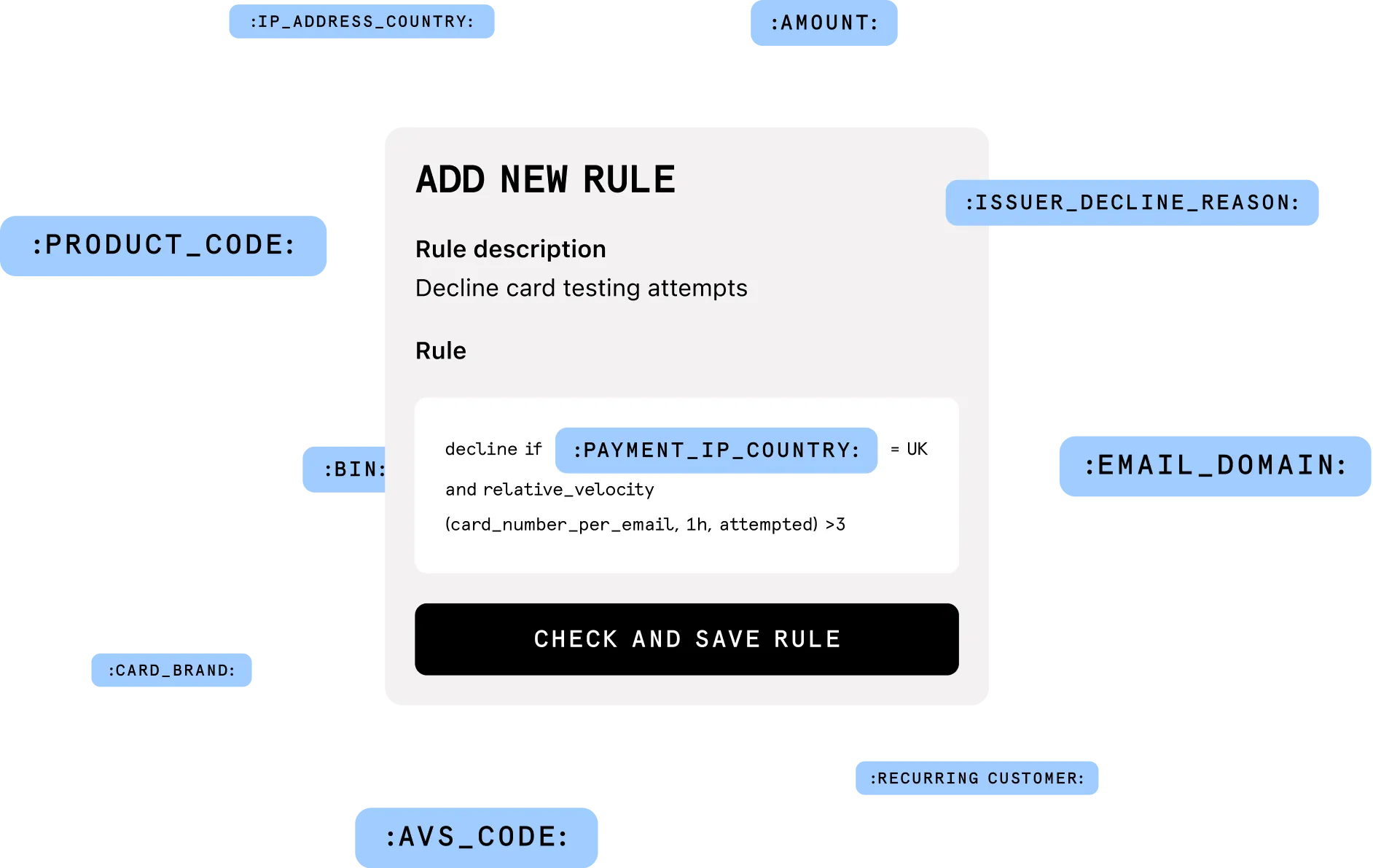
This control does come at a greater administrative cost, but if you’re using both systems, you can maintain full control while allowing the machine learning model to do much of the heavy lifting of data review and analysis. This frees you up to deal with those fringe cases that require more human insight, improving your overall accuracy while reducing friction in the customer payments journey. Take a scalpel to the malignant fraud threat rather than a sledgehammer
Protect yourself against the lagging nature of machine learning models
The scale that machine learning systems can achieve is vital as the payment fraud threat continues to grow, but it takes time for them to reach a high level of effectiveness. They first have to be trained on millions of data points, and it can take anything from one to three months before your model is up to date with all the latest fraud trends.
But when machine learning and rules are used in combination, time to effectiveness is reduced and you can start developing and iterating fraud fighting rules from the get go.
How Checkout.com helps merchants fight fraud
It’s never been more important to implement a fraud fighting solution that you know you can rely on. Checkout.com’s Fraud Detection combines the scale and efficiency of machine learning with the control and transparency of a rules-based system, and, best of all, it's built right into the platform. That means that, from the moment you start taking payments, Fraud Detection is working hard to protect your business without the need for additional integrations.
With Fraud Detection, you’ll get access to a library of useful rules and industry templates, which you can make minor edits to, providing powerful fraud prevention straight out of the box. You’ll also be able to test your rules and utilize our full suite of granular analytics and reports.
If you upgrade to Fraud Detection Pro, you have maximum flexibility over your risk setup. That includes customizable machine learning thresholds, a comprehensive set of rules, the ability to send custom data, and customer segmentation. No more black box problem: each transaction is given a precise risk score and approved or denied based on your thresholds, giving you full control and visibility over each decision.
Speak to a member of our team to find out how you can protect your revenue while improving acceptance with Checkout.com’s Fraud Detection Pro.